Connecting Industrial Sites in Real Time with AI Digital Twin
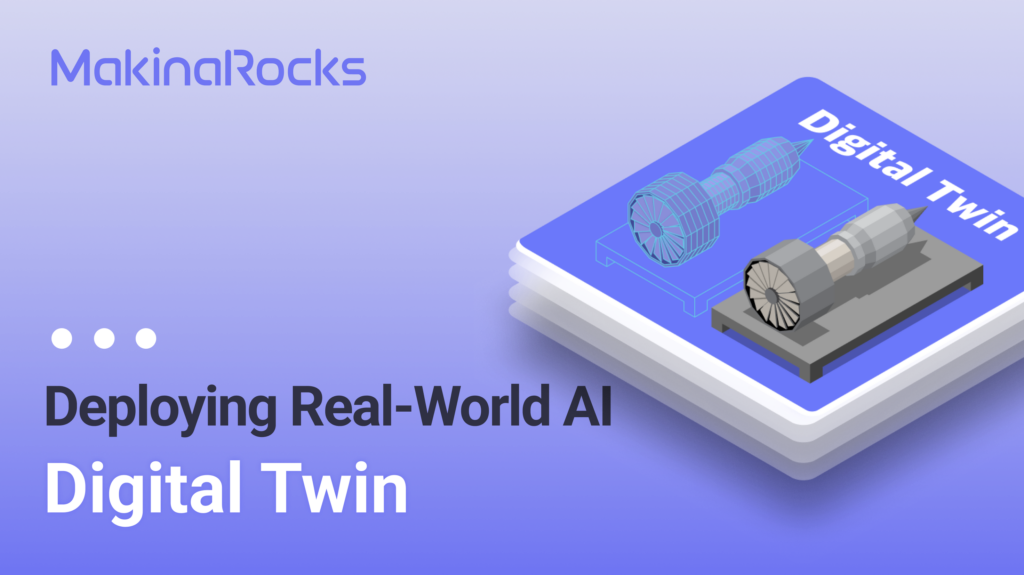
Connecting Industrial Sites in Real Time with AI Digital Twin
Can AI outperform humans? For years, this question remained unanswered—until 2016, when AlphaGo made history by defeating Lee Sedol. AlphaGo, an AI program developed by Google DeepMind, utilized deep learning and reinforcement learning to master the complex game of Go. In March 2016, it triumphed over South Korean 9-dan professional Go player Lee Sedol, winning 4 games to 1, demonstrating the true potential of AI to the world.
The real world is far more complex than Go
Go is known for its immense complexity, with an estimated 10170 possible outcomes—more than the number of atoms in the universe. AlphaGo, leveraging vast computational power, learned and refined its strategy in real time. However, the Go board is a highly controlled environment, with all variables known and finite. In such a setting, AlphaGo’s victory was almost inevitable.
But the real world is far more complex than a Go board. AlphaGo was trained on high-quality, publicly available data from world-class players, optimizing its performance within a structured environment. In contrast, the challenges in the real world are much more unpredictable.
Take the complexity of a manufacturing floor as an example. Variables like temperature, humidity, noise, equipment wear, and scheduling all interact, influencing machine performance and even the timing of part replacements. Additionally, variations in worker skills and changing conditions add to the dynamic nature of the production environment. This complexity is far beyond what AlphaGo was designed to handle.
AI-powered digital twins simulating the complexities of the real world
What if AI could handle the unpredictable environments of industrial sites? Unlike AlphaGo, which operates in a structured game setting, AI trained on real-world data can learn to manage a broad range of variables. In industry, the ongoing challenge is maximizing efficiency and productivity. However, applying AI directly to functioning processes carries risks.
Industrial sites are intricate systems where countless variables interact. If AI models aren’t rigorously validated in real-world scenarios, they could fail unpredictably, potentially interrupting processes or compromising product quality. That’s why a cautious, methodical approach is crucial when implementing new technologies like AI. Testing AI in real-world environments without proper simulation is impractical and risky.
AI-powered digital twins simulate real-world complexities, allowing companies to test AI models in a risk-free environment before live deployment. MakinaRocks has developed its own multi-step simulation dynamics model, creating a digital twin that replicates industrial environments, including processes, equipment, products, and operational conditions. This enables rapid validation and optimization of AI models for tasks like prediction, optimization, and predictive maintenance, ensuring safer innovation without disrupting current operations.
Optimizing, predicting, and preventing with digital twins
Illustration of MakinaRocks’ AI-powered digital twin
In the industrial world, AI-powered digital twins are transforming productivity, operational efficiency, and risk management. While traditional equipment simulators provide valuable insights, they often fall short of capturing real-time changes in process conditions. MakinaRocks’ digital twin overcomes this limitation by offering real-time monitoring of both equipment and process conditions, as well as external factors and operator behavior. This creates a highly accurate and dynamic simulation.
Unlike basic simulators that predict only the next step (single step), MakinaRocks’ digital twin can forecast mid- to long-term (multi-step) state changes, offering a deeper understanding of future developments. By incorporating observation variables, internal and external conditions, and control inputs from real industrial data, the digital twin enables testing of multiple control scenarios. Combined with advanced AI models, this technology effectively addresses complex industrial challenges such as preventing critical failures, optimizing control systems, and improving production schedules.
Digital twins in action for industrial innovation
How are digital twins transforming industries? Let’s look at a practical example in 🔗 energy efficiency for an electric vehicle's air conditioning system. By providing current state data, target temperature, and desired energy efficiency, a reinforcement learning agent calculates control values for the system’s actuators. These values are then fed into the AI-powered digital twin, which simulates the predicted temperature and energy efficiency. The results are compared to the target, and the agent is rewarded based on how closely the goals are met. Through continuous feedback, the agent optimizes control values, ultimately achieving the desired temperature and efficiency.
Beyond electric vehicle air conditioning, MakinaRocks has applied this approach across multiple domains, including 🔗 tuning parameters of industrial machines, 🔗 optimizing temperatures in steel furnaces, and automating waste incinerator operations. In fact, more than 5,000 AI models deployed by MakinaRocks are being validated and used in real-world digital twins, providing precise, AI-driven insights that mirror actual conditions.
Curious about how to leverage AI for real business impact in the complex, unpredictable industrial landscape? Click the banner below to contact us. We’re here to help you turn possibilities into reality.