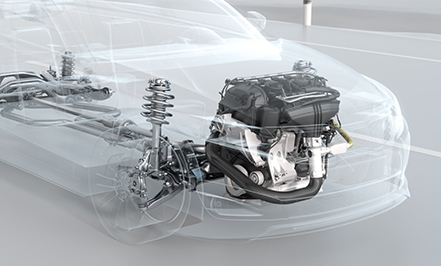
- Automobile
- Optimization
EV HVAC Control Optimization: Energy Use Cut by 10%
Create an AI simulator using real-world electric vehicle data to optimize vehicle energy control with a lightweight model for enhanced computational speed.
READ MOREAs electric vehicles (EVs) become increasingly commercialized, the demand for precise prediction of lithium-ion battery (Li-ion battery) lifespan has intensified. Efficient battery lifecycle management is challenged by the scarcity of comprehensive data, given that few EVs have been driven to the extent of their battery's warranty life. Traditional battery life predictions, which rely on basic criteria like vehicle and battery types, have proven inaccurate. This has heightened the need for a more personalized approach to battery life prediction, one that takes into account real-world driving patterns.
Want to learn more about this use case?
Get in touch with our industrial AI experts.